

.jpg)

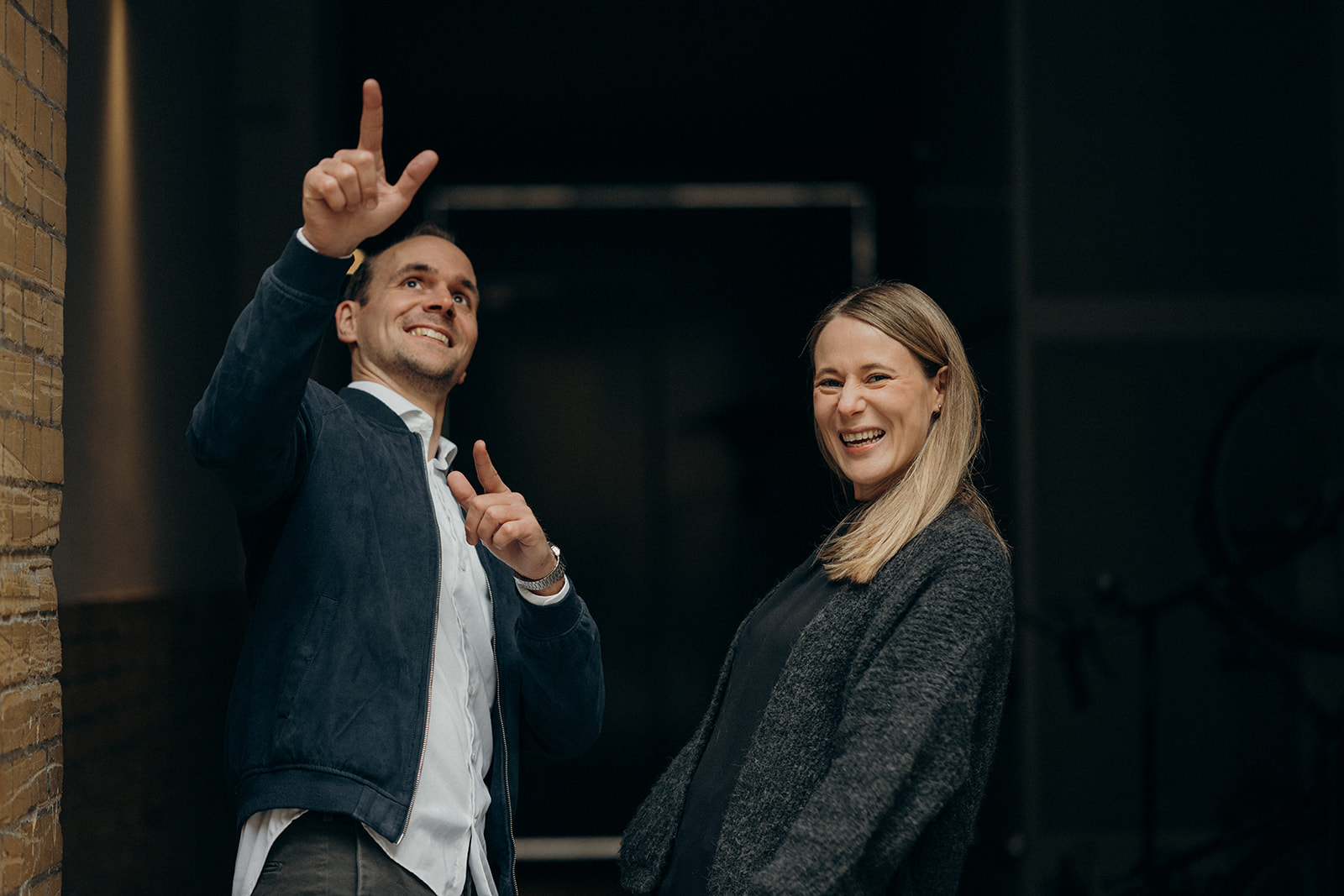

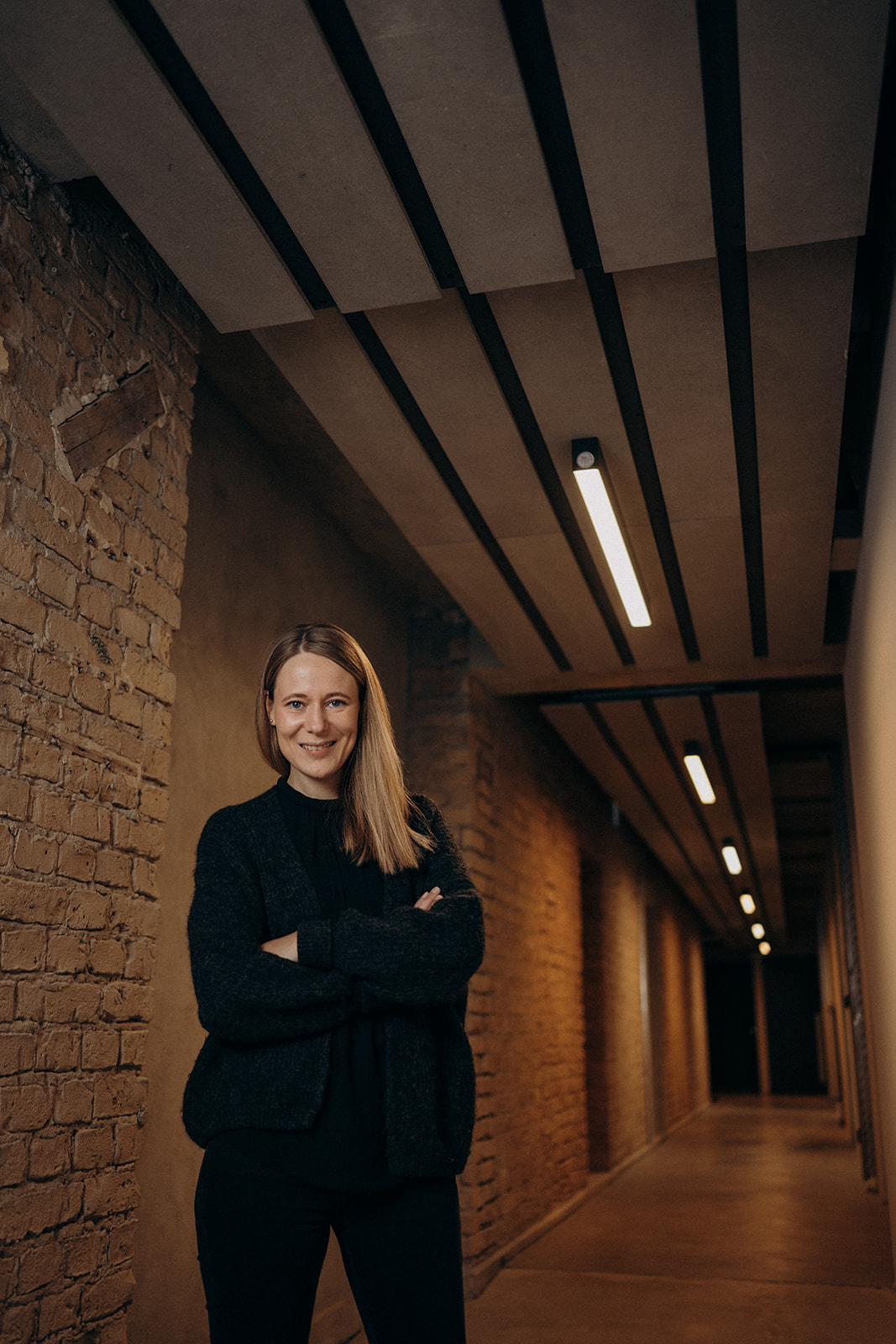



Hasty is a labellling automation platform that aims to automate over 70% of the work needed to train an AI model and experience much faster labeling, be more confident in the data, and deliver successful projects, without the heavy lifting or up-front resources needed before. The tool is available for free – check it out at hasty.ai!
I led the design of this platform and worked on this project for over a year: full-time in the beginning, and part-time later on.
I worked alongside a User Researcher, Data Scientist, Business Development Manager, two Frontend Engineers, and a Backend Engineer.
While applying deep learning to quality assurance tasks in manufacturing at wattx we faced the problem that some use cases required a lot of time to prepare datasets for training purposes — even simple bounding box annotations required hours of “boring” work. We were searching for alternative tools to automate this work, but none had the desired performance or were too expensive. First, we tried to extend existing tools using APIs, but we only got integration problems. Then we tried to hire working students or use crowdsourced platforms, which also took a few days to label a dataset — with suboptimal accuracy. Thus, we decided to build a tool, that fits our requirements.
So when the time of our company-wide hackathon came, my colleague decided to create a prototype that would learn from you on the go, and with the help of machine learning, you would be able to do it faster. After the hackathon, we wrote a blog post, which received a lot of attention, and since wattx is a company builder, we decided to give this project a chance and put together a small team to work on an MVP.
My first product research and design roadmap looked like this:
Designs that came out of the hackathon:
Our early research was mainly focused on Automation Level 1 (please see this article to read more about automation levels). We hypothesized that we had to provide our users with the best possible manual labeling tools so that they could label the first set of images in a speedy manner but not sacrificing the quality. This was confirmed through the first set of interviews and tests.
The defined problem statement was
""
My main goal as a Product Designer was to come up with a smart and familiar way for the users to label the first 10-15 images. As an inspiration, I referred to the tools like Adobe Photoshop and Illustrator.
Here is an overview of the first set of manual tools that we came up with:
magic wand
After the first phase was finished, we focused on AI tools implementation:
We made Hasty Freemium so that users can do a pilot or proof of concept for free and only need to start paying when taking a project into production. Further, we gave users full access to all the AI assistants before having to pay. Our research distinctly showed that this alone could be a great differentiating factor against our competitors.
This meant that my main focus as a designer was to bring first-time users through a funnel of manual labeling to automated labeling as quick as possible because that's where the fun happens!
My initial designs failed miserably after the first (qualitative) user test. The problem was that most of the people understood the concept of tools like Photoshop and Illustrator, but couldn't understand what and how long they needed to go from manual to automated labeling because the set of tools wasn't available for them yet. I solved it firstly by visually drawing a clear distinction between manual and automated sets of tools, and secondly, by providing context menus and notifications that informed the user about the availability of the automated tools.
Before:
After:
Hasty was founded in 2019, and has raised $3.7 million in a seed round led by Shasta Ventures
By november 2019, hasty had 47 alpha users who helped improve hasty and shaped it's future.
In the first year after going live, Hasty claimed around 4,000 users, a fairly even mix of corporations, universities, startups, and app developers that span just about every industry. Hasty also has three of the top 10 German companies in logistics, agriculture, and retail using it.
In 2022, Hasty.ai revenue run rate hit $1.3M. Later that year it was acquired by coudfactory.com.